The AI workload super cycle represents a profound transformation in the technology landscape, underpinned by cutting-edge GPU hardware innovation and a surging demand for AI-driven tasks. Let’s explore the intricate dimensions, emerging trends, and multifaceted dynamics of this rapidly evolving domain.
The evolution of the AI workload super cycle is closely tied to the catalyst of GPU hardware innovation, with Nvidia at the forefront. First we’ll look at how these innovations have set the stage for the super cycle.
GPU hardware innovation: The catalyst
The spark that ignited the AI workload super cycle can be traced back to pioneering GPU hardware innovations, with Nvidia at the forefront. Nvidia's GPUs, particularly those based on the Ampere architecture, have become the de facto standard for AI workloads. Their parallel processing prowess, high memory bandwidth, and specialized hardware for AI tasks have made them indispensable in the field. The introduction of tensor cores, specifically designed for accelerating machine learning workloads, has been a game-changer, drastically reducing training times for deep neural networks.
But hardware alone doesn't define the super cycle; it's also driven by the surge in demand for AI-driven tasks. To fully appreciate the implications of this demand, let's explore the initial surge of AI training workloads.
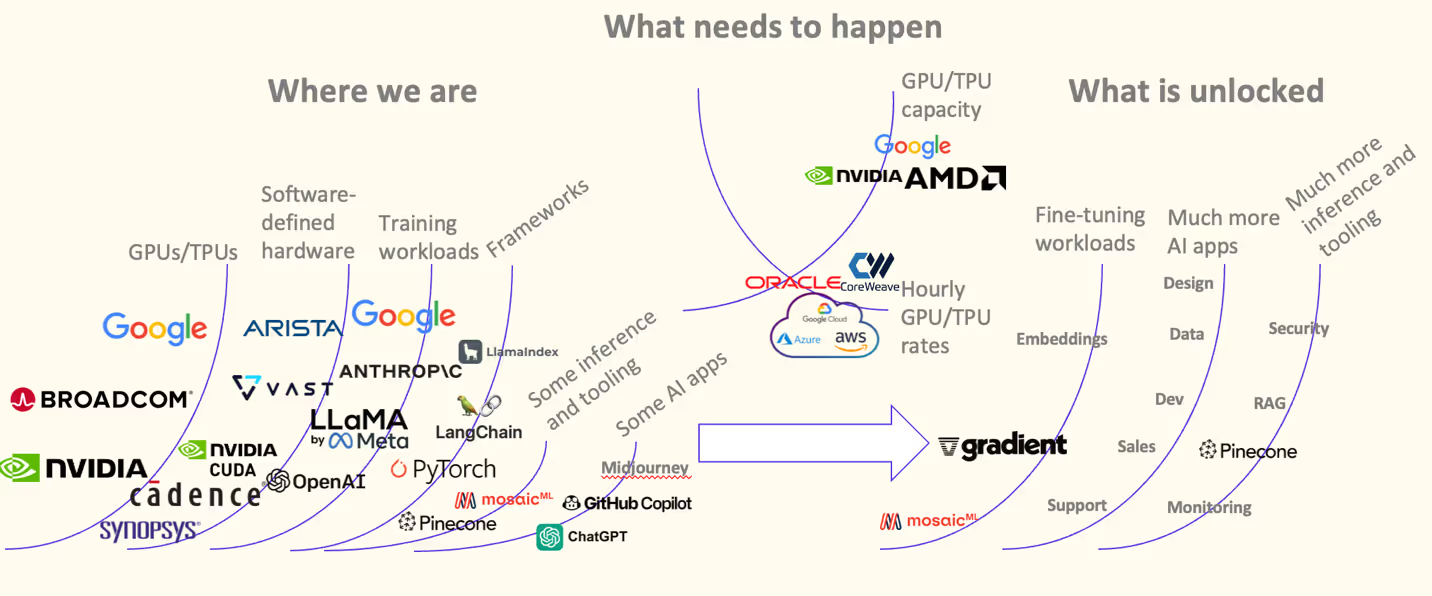
Training workloads: The initial surge
The initial wave of the AI workload super cycle predominantly revolved around training tasks. Prominent examples include OpenAI's GPT-3, Google's Gemini, Anthropic's research efforts, and Llama-2's large-scale language model. Training neural networks is a resource-intensive process that requires massive computational power and large datasets. To harness the full potential of GPUs, software-defined hardware and domain-specific architectures (DSAs) have emerged as critical innovations. DSAs allow for the customization of hardware to match the specific requirements of AI workloads, leading to significant gains in energy efficiency and performance.
The synergy between GPU hardware and software frameworks like CUDA, PyTorch, TensorFlow, and MXNet has empowered practitioners to train ever-larger models, leading to breakthroughs in natural language processing, computer vision, and reinforcement learning. However, the imperative of lowering GPU costs remains a pervasive challenge.
The imperative of lowering GPU costs
While AI workloads have witnessed remarkable progress, the pervasive challenge of high GPU costs remains a formidable barrier to widespread adoption. OpenAI's experience, marked by negative gross margins due to significant GPU usage costs, underscores the urgency of addressing this challenge.
Efforts to lower GPU costs are multifaceted. Research in hardware efficiency, such as mixed-precision training and model sparsity techniques, aims to reduce the computational requirements of AI workloads. Innovations in cloud-based GPU services, like AWS's EC2 instances with GPU support, offer more flexible and cost-effective options for scaling AI projects.
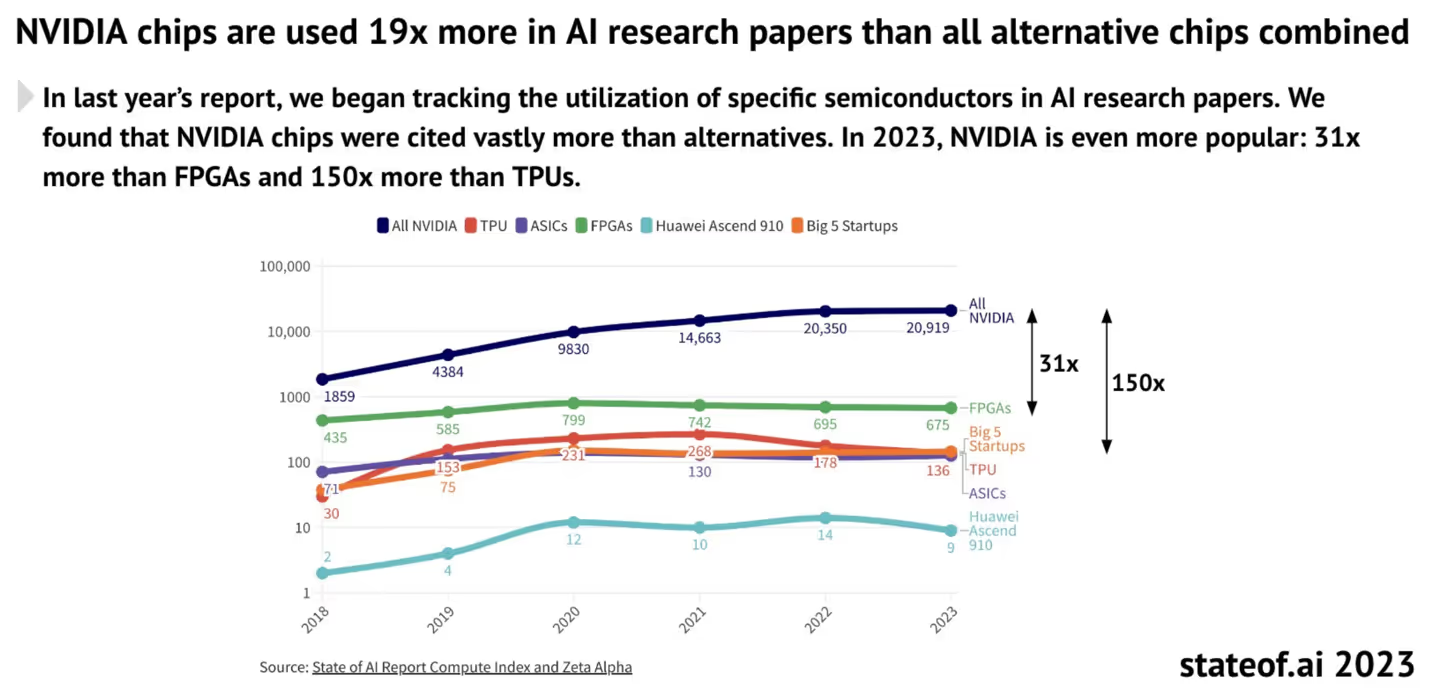
Additionally, collaborations between hardware vendors and cloud providers are driving the development of specialized AI hardware solutions, optimized for cost-effective performance.
Now that we've touched upon the challenges, let's look closer at how the evolving AI landscape is shaping the super cycle.
The evolving AI landscape
The AI workload super cycle not only drives technological advancements, but also reshapes the broader landscape of AI applications. GPU providers play a pivotal role in shaping the direction of AI, akin to the influence exerted by cloud providers in previous technology cycles. As AI becomes increasingly integrated into industries ranging from healthcare and finance to autonomous vehicles and entertainment, the demand for specialized hardware solutions and software frameworks continues to soar. This landscape shift underscores Nvidia's ascendancy in the AI sphere.
Nvidia's ascendancy in the AI sphere
Nvidia's ascendancy in the AI realm has been nothing short of spectacular. The company's market capitalization has surpassed the trillion-dollar mark, reflecting the pivotal role it plays in enabling AI innovation. Contracts for GPU inventory have reached unprecedented levels, cementing Nvidia's position as a juggernaut in the GPU and AI infrastructure arena.
But historical context tells us that exuberant investments in infrastructure often accompany technology super cycles. Let's delve into this aspect to provide a broader perspective.
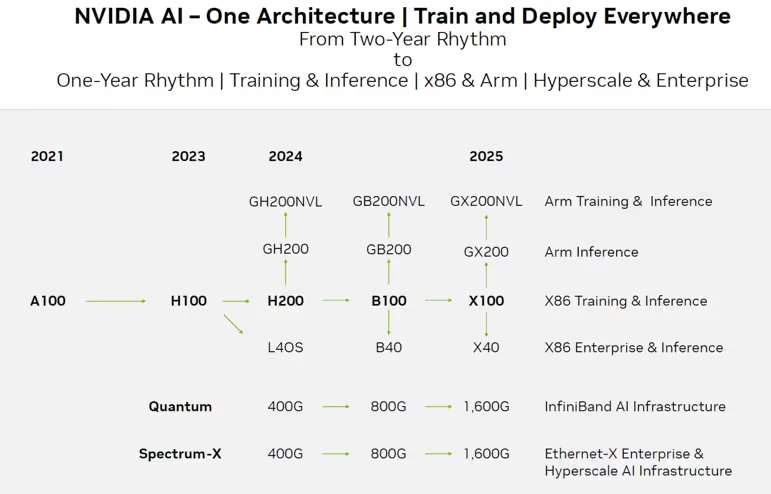
Infrastructure overbuild: Historical perspective
Historically, technology super cycles have been characterized by exuberant investments in infrastructure. The current GPU super cycle draws parallels to past phenomena, such as the telecom super cycle, where infrastructure investments ultimately led to a proliferation of consumer applications. This historical context highlights the potential for AI to transition from a specialized field to a pervasive and transformative force across industries.
As we move forward, it's essential to recognize that AI workloads are evolving beyond training.
The state of AI workloads
While a substantial portion of AI workloads continues to focus on training, there is a discernible shift towards inference workloads. Entities like Coreweave predict that inference will soon dominate the AI workload landscape. Inference tasks, which involve deploying trained models to make real-time predictions or decisions, are integral to applications like natural language understanding, recommendation systems, and autonomous vehicles. The rise of edge computing, where AI processing occurs on local devices, further underscores the growing importance of inference workloads.

This shift in focus opens up new opportunities for innovation, particularly concerning the convergence of unstructured data and AI.
Unstructured data and AI: The convergence
AI workloads, particularly those centered on large language models (LLMs), heavily rely on unstructured data. Converting unstructured data into structured context is a critical challenge in AI. Innovations from companies like Vast Data, which offer solutions for infusing structured context into unstructured data, are poised to revolutionize AI workloads. This convergence of structured and unstructured data processing not only enhances the efficiency of AI models but also opens up new avenues for insights and applications.
As the landscape becomes increasingly competitive, it's crucial to address the challenges and competition that Nvidia and other players face.
Challenges and competition
Nvidia's preeminence in the AI hardware arena is not without its challenges. Competitors like AMD are intensifying their efforts to capture a share of the AI hardware market. AMD's high-performance GPUs and strategic partnerships may pose a formidable threat, particularly in the domain of inference workloads. Additionally, tech giants like Google are investing heavily in developing custom AI hardware, seeking to reduce their dependence on external GPU providers.
Furthermore, the sustainability of AI workloads, in terms of both energy consumption and hardware production, is a growing concern. Efforts to design energy-efficient AI hardware, as well as initiatives to promote responsible AI development, are gaining traction. The competition and collaboration among hardware vendors, cloud providers, and research institutions will continue to shape the future of AI hardware and workloads.
Developer response: Navigating GPU costs
Developers and organizations are proactively exploring strategies to navigate the challenges posed by escalating GPU costs. These strategies include adopting smaller and more efficient models, leveraging open-source alternatives to proprietary frameworks, and forming strategic partnerships with hyperscale cloud providers. The assessment of the return on investment (ROI) for GPU usage assumes critical significance in this landscape, prompting developers to make informed decisions about the size and complexity of AI models and the choice of hardware infrastructure.
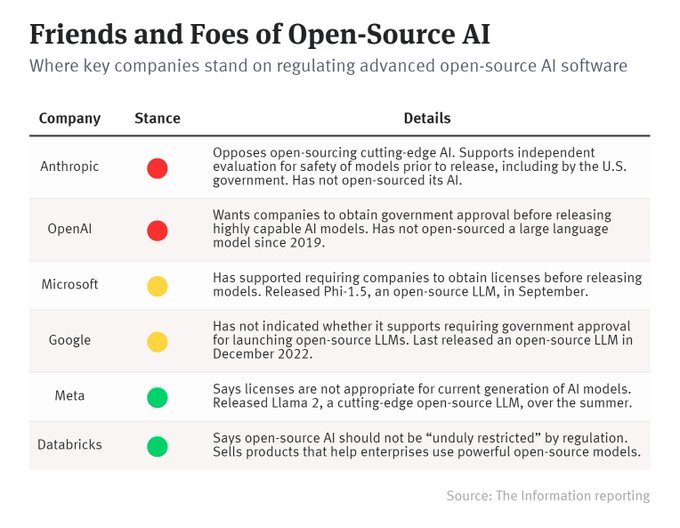

Forging ahead
The AI workload super cycle is not merely a technological phenomenon; it is a seismic shift that is reshaping the core of the technology industry. Nvidia's leadership in this space, driven by relentless innovation and strategic partnerships, is emblematic of the transformative power of AI workloads. Despite formidable challenges and intensifying competition, the future of AI workloads holds immense promise, brimming with opportunities for innovation, discovery, and societal impact. As the super cycle continues to unfold, it will redefine the boundaries of what AI can achieve, unleashing its full potential to drive progress and transformation across diverse domains. The journey ahead promises to be as exhilarating as it is challenging, as we collectively explore the uncharted territories of AI's capabilities and applications.